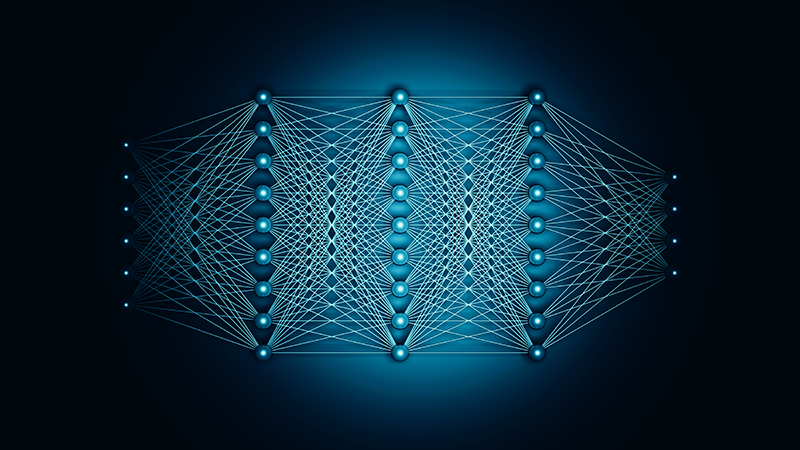
Artificial Neural Networks
by Bryant Cruse | Nov 6, 2017 | Narrow AI
Narrow AI’s Dark Secrets
Articles about AI are published every day. The term “AI” is used in a very narrow sense in the majority of these articles: it means applications based on training artificial neural networks under the control of sophisticated algorithms to solve very particular problems.
Here is the first dark secret: This kind of AI isn’t even AI. Whatever this software has, the one thing it lacks is anything that resembles intelligence. Intelligence is what distinguishes us from the other animals as demonstrated by its product: knowledge about the world. It is our knowledge and nothing else that has made us masters of the world around us. Not our clear vision, our acute hearing, or our subtle motor control, other animals do that every bit as well or better. The developers of this technology understand that and so a term was invented some years ago to separate this kind of program with real AI; Narrow AI which is in use in contrast to Artificial General Intelligence (AGI) which is the kind that processes and creates world knowledge.
Here’s the second dark secret. The machine learning we have been hearing about isn’t learning at all in the usual sense. When a human “learns” how to ride a bicycle, they do so by practicing until the neural pathways that coordinate the interaction of the senses and muscles have been sufficiently established to allow one to stay balanced. This “neural learning” is clearly very different than the kind of “cognitive learning” we do in school which is based on the acquisition and refinement of knowledge. Neural learning cannot be explained and cannot be unlearned, no abstract knowledge of the world is produced. A circus bear can ride a bike but we don’t say it is intelligent because of that.
The third dark secret: We don’t understand how the sophisticated algorithms that control the training of these networks actually work. This fact is probably at the root of the fear that Artificial Intelligence may someday escape human control.
But if narrow AI is not real AI why is it considered AI at all? It is because of the hope that someday these narrow techniques may be extended to become the real thing and real AI is a very exciting, world-changing prospect. That makes these current efforts more glamorous to the general public, easier to hype, and easier to attract funding. But the hype has gone too far and has engendered a growing expectation that real AI is just around the corner and we had better be prepared for its civilization-changing effects.
Today, the AI community is starting to back-pedal big time. We are seeing a growing admission coming from both the big tech companies and academia that the hope that these techniques can be evolved into real AI is, if not totally forlorn, certainly not so imminent as the general public and the media have been led to believe.
Will the Future of AI Learning Depend More on Nature or Nurture?
Yann LeCun, a computer scientist at NYU and director of Facebook Artificial Intelligence Research.
“None of the AI techniques we have can build representations of the world, whether through structure or through learning, that are anywhere near what we observe in animals and humans”
Facebook’s head of AI wants us to stop using the Terminator to talk about AI
“We’re very far from having machines that can learn the most basic things about the world in the way humans and animals can do.”
“… in terms of general intelligence, we’re not even close to a rat.”
“The crucial piece of science and technology we don’t have is how we get machines to build models of the world.”
“The step beyond that is common sense, when machines have the same background knowledge as a person.”
Inside Facebook’s Artificial Intelligence Lab
“Right now, even the best AI systems are dumb, in the way that they don’t have common sense.”
“We don’t even have a basic principle on which to build this. We’re working on it, obviously, We have lots of ideas, they just don’t work that well.”
Why Google can’t tell you if it will be dark by the time you get home — and what it’s doing about it
Emmanuel Mogenet, head of Google Research Europe:
- “But coming up with the answer is not something we’re capable of because we cannot get to the semantic meaning of this question. This is what we would like to crack.”
- He explained that Google needs to try and build a model of the world so that computers know things like …
- “I’ll be honest with you, I believe that solving language is equivalent to solving general artificial intelligence. I don’t think one goes without the other. But it’s a different angle of attack. I think we’re going to push towards general AI from a different direction.”
Microsoft CEO says artificial intelligence is the ‘ultimate breakthrough’
Satya Nadella, Microsoft CEO
“We should not claim that artificial general intelligence is just around the corner,”
“We shouldn’t over-hype it.”
“Ultimately, the real challenge is human language understanding – that still doesn’t exist. We are not even close to it…”
“The Real Trouble With Cognitive Computing”
Jerome Pesenti, former vice president of the Watson team at IBM.
“When it comes to neural networks, we don’t entirely know how they work, and what’s amazing is that we’re starting to build systems we can’t fully understand. The math and the behavior are becoming very complex and my suspicion is that as we create these networks that are ever larger and keep throwing computing power to it, …. (it) creates some interesting methodological problems.”
Read More
Calm down, Elon. Deep learning won’t make AI generally intelligent
Mark Bishop, professor of cognitive computing and a researcher at the Tungsten Centre for Intelligent Data Analytics (TCIDA) at Goldsmiths, University of London:
It’s this lack of understanding of the real world that means AI is more artificial idiot than artificial intelligence. It means that the chances of building artificial general intelligence is quite low, because it’s so difficult for computers to truly comprehend knowledge, Bishop told The Register.
The Dark Secret at the heart of AI.
Joel Dudley leads the Mount Sinai AI team.
“We can build these models,” Dudley says ruefully, “but we don’t know how they work.”
Creative Blocks, Aeon Magazine
David Deutsch, quantum computation physicist at the University of Oxford:
“Expecting to create an AGI without first understanding how it works is like expecting skyscrapers to fly if we build them tall enough.”
“No Jeopardy answer will ever be published in a journal of new discoveries.”
“What is needed is nothing less than a breakthrough in philosophy, a new epistemological theory…”